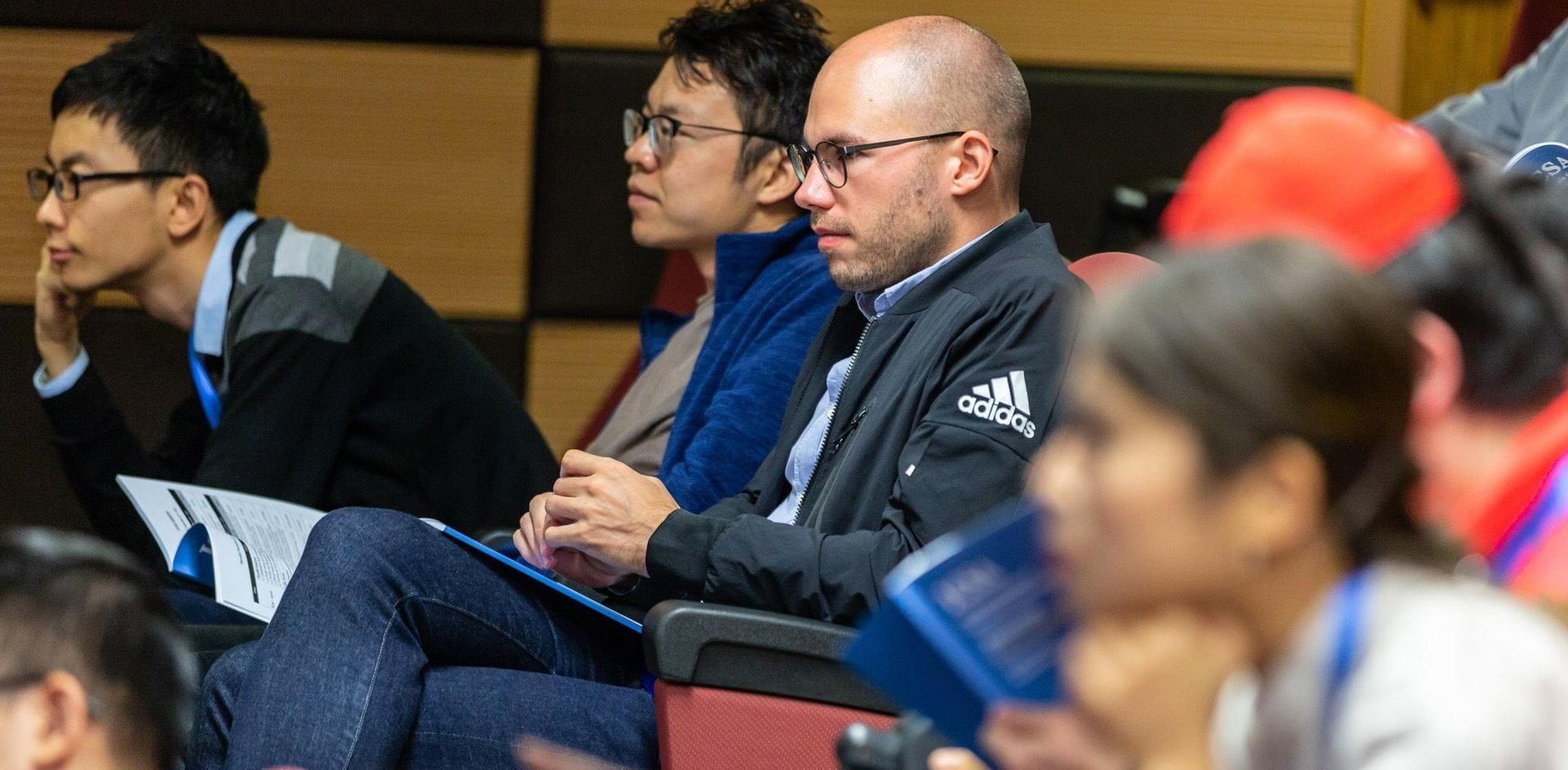
Embedded EthiCSTM @ Harvard Bringing ethical reasoning into the computer science curriculum
AI for Social Impact (CS 288) – Spring 2021
First time reviewing a module? Click here.
Click ⓘ to access marginalia information, such as reflections from the module designer, pedagogical decisions, and additional sources.
Click “Download full module write-up” to download a copy of this module and all marginalia information available.
Module Topic: Ethical Reasoning in AI for Social Impact
Module Author: Samuel Dishaw (drawing on previous work by Marion Boulicault)
Course Level: Primarily Graduate
AY: 2020-2021
Course Description: “The key thrust behind the fast emerging area of “AI for social impact” has been to apply AI research for addressing societal challenges. AI has a great potential to provide tremendous societal benefits in the future. In this course, we will discuss the successful deployments and the potential use of AI in various topics that are essential for social good, including but not limited to health, environmental sustainability, public safety and public welfare. In AI, we have just recently begun to define this area as its own area of research, and we have just recently started understanding that the area includes more than simply providing methodological advances in terms of newer models and algorithms. This course is focused on understanding the latest research in this area, and discussing
foundations. In doing so, we will familiarize ourselves with key open questions in this emerging area of research.” (Course description)
Semesters Taught: Spring 2021, Fall 2022
Tags
- AI for Social Impact (CS)
- optimization (CS)
- fairness (both)
- aggregation (phil)
- social justice (phil)
Module Overview
ⓘ
A word on the first project: social network-based substance abuse interventions consist in pairing youth within a network with other youth in small groups, as a way of promoting positive habits and reducing their substance use. This kind of intervention is commonly used by social workers, and it can be very beneficial. But it can also have the opposite effect from the one intended, namely that of reinforcing substance abuse habits. This effect is called ‘deviancy training’. The aim of the project is to use AI in order to determine how to partition a certain number of at-risk youth into groups, so as to maximize the positive social network effects. The second project is more straightforward: it uses AI to optimize the scheduling and movement of security personnel, with the objective of deterring fare-evaders.
The module discusses the ethical implications of two real-life projects from within the area of AI for social impact. The first project uses AI to optimize social networks in the context of substance abuse interventions. The second project uses AI to combat fare evasion on the Los Angeles public transit system. The overall aim of the module is to illustrate how ethical issues can arise at different stages of an AI for Social Impact project, and how students can address these ethical issues in their own project planning.
Connection to Course Technical Material
ⓘ
The course culminates with students designing their own AI for Social Impact project. As part of these projects, students are asked to identify some ethical challenges and pitfalls of their project and explain how their project would address these challenges. The two case studies discussed in this module are a way for students to see what such an ethical analysis would look like.
AI for social impact naturally raises a host of questions about who should benefit, how those benefits should be distributed, and, more fundamentally, what social goods are worthy of being promoted in the first place, and under what conditions.
Goals
Module Goals
- Introduce a distinction between two moral methods for allocating resources.
- Apply these two methods to an optimization problem (peer networks for substance abuse prevention).
- Discuss the case of using AI to combat fare evasion, and whether that project promotes a social good that is worth promoting.
- Distinguish different stages of project development at which ethical issues might arise.
Key Philosophical Questions
ⓘ
The questions under heading “1” are discussed with respect to the case of social network-based substance abuse prevention. The basic problem being: when using AI to determine how to form groups, the optimal group partition is in which the youth that are most at-risk are all put in a group together, essentially so as to ‘isolate’ other participants from their bad influence. This partition is optimal because it has the highest total expected benefits aggregated across individuals. Students discuss a hypothetical choice between a partition of this kind, and one which does less aggregate good overall, but gives the most at-risk youth the best chance at recovery.
The questions under heading “2” are elicited from a discussion of the case of using AI to combat fare evasion. In that discussion, students are invited to consider whether there are instances in which it is acceptable to free ride , whether someone’s having a general policy of never paying their fare could be justified, and what this means for whether reducing fare evasion is a goal we should pursue.
- How should we choose between different ways of allocating a limited resource? Should we choose the allocation that has the highest total expected benefits across individuals, or should we, in some sense, give priority to the worst off?
- Do users of public transit owe it to each other, as a matter of fairness, to pay their fare? And does the answer to this question depend on whether the society of which these users are a part is just or unjust?
Materials
Key Philosophical Concepts
ⓘ
The notions of aggregation and pairwise comparison correspond to the two moral methods that were applied to the case of social networks for substance abuse prevention. Rawls’ notion of a duty of fair play is used to provide some ethical motivation for the project of combatting fare evasion.
Shelby’s critique of Rawls via the notion of the limits of tolerable injustice is used to put pressure on the idea that all users have a duty of fair play to others to pay their fare, and thereby to cast doubt on whether reducing fare evasion is a social good worth pursuing.
- Aggregation
- Pairwise Comparison
- The Duty of Fair Play
- Limits of Tolerable Injustice
Assigned Readings
ⓘ
Hirose and Kamm put forward competing approaches to the question of how to allocate scarce resources. Shelby provides a critique of the idea that those disadvantaged by social injustice have a moral duty to obey the law just for its own sake, or a moral duty to cooperate with their co- citizens even in cases when their failure to cooperate doesn’t harm anyone.
- Shelby, T. (2007). “Justice, Deviance, and the Dark Ghetto”, Philosophy and Public Affairs.
- Hirose, I. (2014). Moral Aggregation, (selections).
- Kamm, F. (2020). “Moral Reasoning in a Pandemic”. The Boston Review.
Implementation
Class Agenda
- Two Moral Methods
- Case #1: Social network based substance abuse prevention
- Case #2: Fare Evasion
- ‘Fair Play’ and Social Justice
- Ethical Reasoning from data to deployment
Sample Class Activity
ⓘ
Students engage well with this activity, and come up with very creative answers in response to (ii), which departs from both the strict pairwise comparison and aggregation method. For instance, one group of students proposed that the sorting algorithm should ensure that each individual has some threshold probability of recovery, and then optimize the aggregate expected number of non-users only once that threshold is reached.
In the module, students are told that the expected number of non- users in Isolate was greater than in Distribute (6.3 against 4.8). It might be worth withholding that information and letting students work out the details themselves first while they go about answering question (i).
Students discuss the merits of two different group partitions for network-based substance abuse prevention, first in small groups and then together in open discussion. The two group partitions were Isolate: {0, 0, 0}, {.9, .9, .9}, {.9., .9, .9, .9}, and Distribute: {.3, .5., .5}, {.3, .5., .5}, {.4, .6, .6, .6}. Each number corresponds to the probability, for a given individual, of being a non-user at the end of the intervention. Isolate groups the heaviest users together, thus giving a greater chance of recovery for the light users but giving no chance of recovery for the heaviest users. Distribute places one of the three heavy users in each group, thereby giving them a better chance of recovery, but also lowering the chances of recovery for the lighter users. Students are tasked with thinking through two questions:
- Which partition does the aggregative moral method deem to be better, and which partition does the pairwise comparison method deem to be better?
- What should a sorting algorithm optimize for in this case?
Module Assignment
ⓘ
Students answer these questions as part of a “broader impact statement” within their written proposal for an AI for Social Impact project.
“Ethical challenges and pitfalls: When applying interventions, it is feasible that not all stakeholders benefit equally. Or there may be potential harms due to the interventions. What are some steps to ensure that we think through these challenges? What downstream effects should we watch out for?”
The post-class assignment for this module was integrated into student reports for final projects. Alongside answers to technical questions about the AI systems they designed, students were asked to write a statement of the ethical impacts of their projects within the Value Sensitive Design framework.
Lessons Learned
- Students really like the pairwise comparison tool as a way to formalize an alternative to aggregative views such as utilitarianism.
- Students also liked the idea of giving priority to the worst off, since it had application in both of the case studies we looked at.
Except where otherwise noted, content on this site is licensed under a Creative Commons Attribution 4.0 International License.
Embedded EthiCS is a trademark of President and Fellows of Harvard College | Contact us