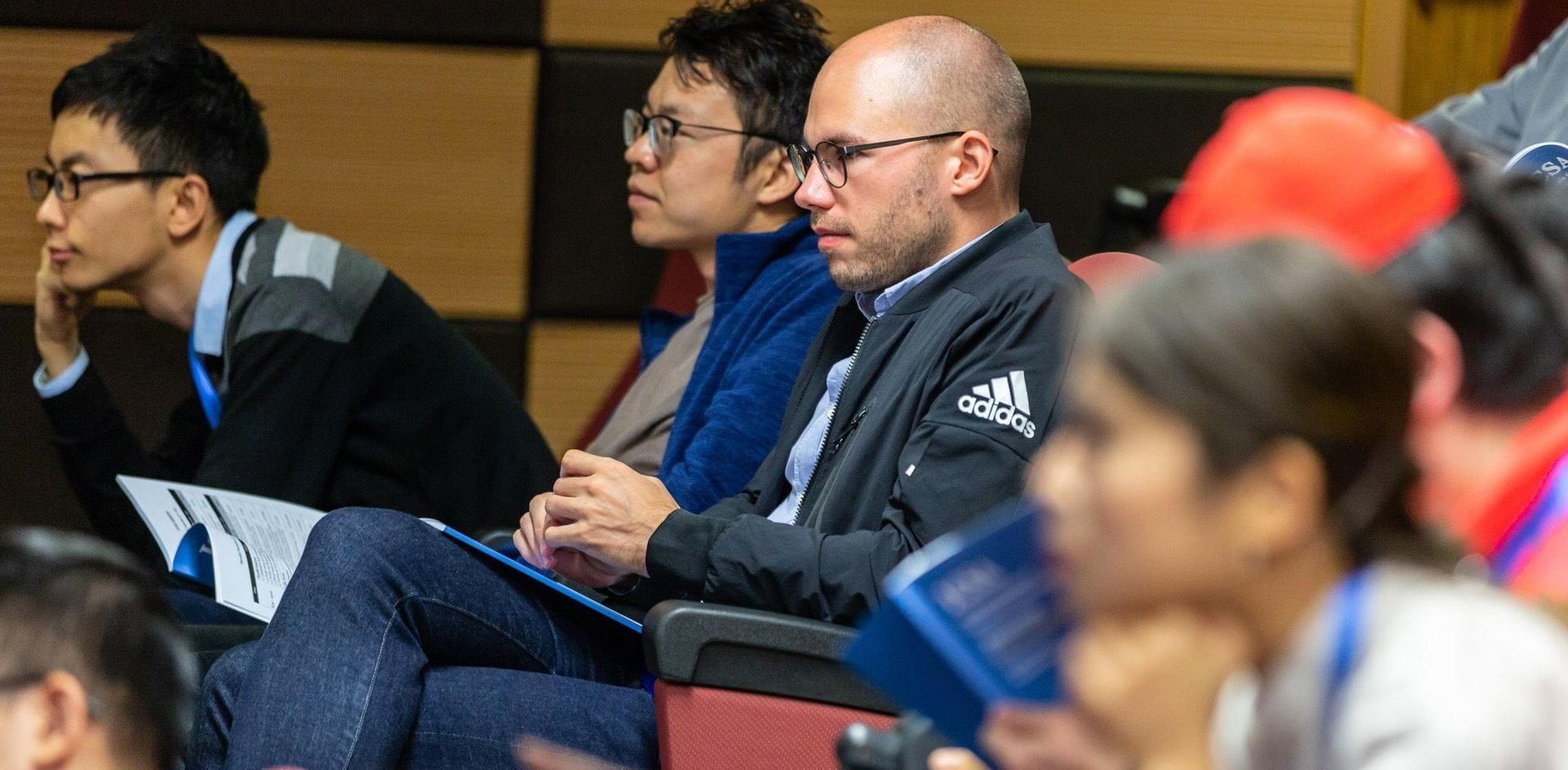
Embedded EthiCSTM @ Harvard Bringing ethical reasoning into the computer science curriculum
Research Topics in Human-Computer Interaction (CS 279r) – 2022 Fall
First time reviewing a module? Click here.
Click ⓘ to access marginalia information, such as reflections from the module designer, pedagogical decisions, and additional sources.
Click “Download full module write-up” to download a copy of this module and all marginalia information available.
Module Topic: Stereotypes, Reflection and Transparency
Module Author: Megan Entwistle
Course Level: Graduate
AY: 2022-2023
Course Description: “Students will read and discuss systems HCI papers, i.e., papers published in human-computer interaction and related venues that build and evaluate novel systems, with a focus on systems that work especially well with (or clash against) human cognitive capabilities. Activities will also include lectures on key topics relevant to building and testing systems in HCI. As a final project, students will implement and evaluate components of one or more selected papers and present their findings in writing and orally in a conference-style format, as means to understand more deeply the processes behind systems HCI research.”
Semesters Taught: Fall 2019, Fall 2020, Fall 2022
Tags
- bias (phil)
- stereotypes (phil)
- transparency (phil)
- human-computer interaction (CS)
- systems with AI (CS)
- voice assistants (CS)
Module Overview: This module takes up the issue of social bias in human-computer interactions. We discuss ways in which biases and stereotypes can show up in HCI design, why this can be ethically problematic, and how mitigating these biases/stereotypes will often require trade-offs with other HCI design principles (e.g. meeting user expectations). We then interrogate the line of thought according to which technology is a morally neutral mirror, which at worst merely reflects problematic user biases back at users. Pressure is put on this idea from two directions: first, research in social psychology on the phenomenon of stereotype threat provides a model of how merely making a harmful stereotype salient can have the effect of further perpetuating it; second, the case study of Google’s search algorithms is leveraged to show that technologies can perpetuate biases even when those biases are not a reflection of user’s individual or collective attitudes. The module closes by considering the role that a transparency design principle might play in bias mitigation.
Connection to Course Technical Material
ⓘ
The topic was chosen upon instructor request. It fits well with the pedagogical goals of the course, has clear practical import for HCI design, and lends itself to opinionated discussion amongst students who might not otherwise have much background in ethical theory.
In the course, students engage with theoretical frameworks for conducting systems-HCI research, and practice designing user-test systems. To both ends, students read a paper on design guidelines for HCI researchers. One guideline recommends the following: “Ensure the AI system’s language and behaviors do not reinforce undesirable and unfair stereotypes and biases.” The authors of the paper reveal that practitioners find this guideline difficult to interpret and evaluate against particular HCI systems. This difficulty points to a clarificatory and normative role that ethical theorizing can play in connection with HCI design principles. The module is designed to give students some tools for thinking about bias mitigation in relation to their own projects.
Goals
Module Goals
- Identify potential instances of social bias in HCI as well as reasons why this can be harmful.
- Illuminate ways in which independently plausible HCI design principles stand in tension with one another.
- Provide students with tools to critique the idea that HCI systems ultimately cater to user preferences and therefore the emergence of bias is not something for which designers are morally responsible.
- Encourage students to critically reflect on the relationship that users have towards technology.
- Help students anticipate problems of bias/stereotypes in HCI as they develop their own projects.
Key Philosophical Questions
ⓘ
Further questions in the background of discussions include what a healthy relationship between users and technologies should look like, and to what extent designers or companies have a moral responsibility to actively mitigate (as opposed to avoid reinforcing) social bias.
- What are biases/stereotypes, and in which HCI contexts can they be harmful?
- How do different HCI design guidelines come into conflict, and which ought to take priority?
- Is the mere reflection of users’ biased attitudes and stereotypes in HCI morally neutral?
- Ought the technologies used in HCI be transparent to users?
Materials
Key Philosophical Concepts
ⓘ
The distinction between reflection of bias and reinforcement of bias is used as a tool for students to analyze potentially problematic HCI contexts.
For example, students were presented with the case of ElliQ, an AI-enabled voice assistant designed to keep at-home seniors connected and engaged. They were asked to discuss whether it is ethically problematic for the default voice’s gender to be female. Some students expressed the view that the default option reflects societal expectations of which voice types sound comforting or helpful, which is a way of matching user preferences. Other students pointed out that because of the functions that ElliQ performs in the context of a care home, the default female voice will further reinforce stereotypical associations between, say, being a nurse or receptionist and being female.
- Bias and stereotypes
- Reflection vs. reinforcement
- Moral responsibility
- Transparency
Assigned Readings
ⓘ
“Guidelines for Human-AI Interaction” (Amershi et. al.) The HCI design guidelines paper was assigned by the instructor for a session earlier in the semester (prior to the date of the module). The relevant parts were cited during the module presentation.
“What is a stereotype? What is stereotyping?” (Erin Beeghly) Students were asked to read the philosophical paper on stereotypes as a primer on the topic. The author of the paper makes helpful distinctions between various ways that stereotyping can be harmful. The module borrows the author’s characterization of stereotypes.
Algorithms of Oppression, chapter one (Safiya Noble) Noble’s intersectional power analysis of Google’s search algorithms was the basis for the third part of the discussion: critiquing the idea of technology as a morally neutral mirror. Noble explains how commercial pressures behind search engine optimization (SEOs) drive racist and sexist content to the top of search results.
- “Guidelines for Human-AI Interaction” (Amershi et. al.)
- “What is a stereotype? What is stereotyping?” (Erin Beeghly)
- Algorithms of Oppression, chapter one (Safiya Noble)
Implementation
Class Agenda
- Introduce the “mitigate social bias” guideline from the HCI design guidelines paper. Ask students to think about whether it is violated in particular cases.
- Demonstrate potential trade-offs between mitigating bias, on the one hand, and other HCI design goals such as matching relevant social norms, or learning from user behavior, on the other hand.
- Articulate the central idea the module is interrogating: that technology (at worst) simply reflects users’ attitudes and stereotypes back at them.
- Discuss possible harms of stereotype/bias reflection in HCI, drawing on the phenomenon of stereotype threat in social psychology research as a motivating example.
- Raise a question about whose attitudes (the actual users? the predicted user? the collective majority? those in positions of power?) are supposedly being reflected in certain HCI contexts.
- Present the Google search case study and Safiya Noble’s power analysis to call into question the reflection metaphor itself and raise a concern about transparency.
- Conclude with a discussion of the prospects of a transparency guideline for mitigating social bias in HCI.
Class Activity
ⓘ
If the layout of the room allows, the module instructor can discuss examples with groups individually, and then ask groups to share what they came up with to the class at large. By this point in the module, students are able to articulate what other HCI design guidelines might explain the emergence of the stereotype, thus building upon the earlier discussion of guideline trade-offs.
After being presented with the research on stereotype threat, students are asked to form small groups and generate examples of their own in which a similar effect is at play: an interaction with technology makes a certain stereotype salient to the user, as a result of which the user is adversely impacted.
Module Assignment
ⓘ
The purpose of the assignment is to apply the ethical thinking they practiced during the module to their own HCI work. The professor and the module TA decide who makes the evaluations.
As part of their final projects, students are expected to write a one-paragraph reflection on why they think the HCI system they are designing either (i) reflects, (ii) reinforces, (iii) mitigates, or (iv) has no bearing on social bias.
Lessons Learned
Student engagement during the module was very high. Students were enthusiastic about discussing the topic both in small groups and as an entire class. They found it interesting to think about possible harms and trade-offs in particular cases, and were quick to get on board with the starting idea that mitigating social bias should be a goal of HCI researchers.
Three further pedagogical lessons from the module are:
- Students already have an intuitive grasp of what stereotypes and biases are, and why they can be harmful. Discussions of particular examples are more useful than general characterizations.
- The Google Search case study is the most difficult for students to engage with (despite the assigned background reading), in part because the empirical facts grounding Noble’s analysis are quite complex and require distilling to get to the important insights. It might be better to use this case to motivate the problem of bias in HCI, and avoid discussing the particularities of search engine architectures (and their susceptibility to economic pressures).
- When this module runs as a 75-minute course, not much time is left for a discussion of transparency. Future module instructors may wish to cut one of the agenda items (from 5-7 above).
Except where otherwise noted, content on this site is licensed under a Creative Commons Attribution 4.0 International License.
Embedded EthiCS is a trademark of President and Fellows of Harvard College | Contact us