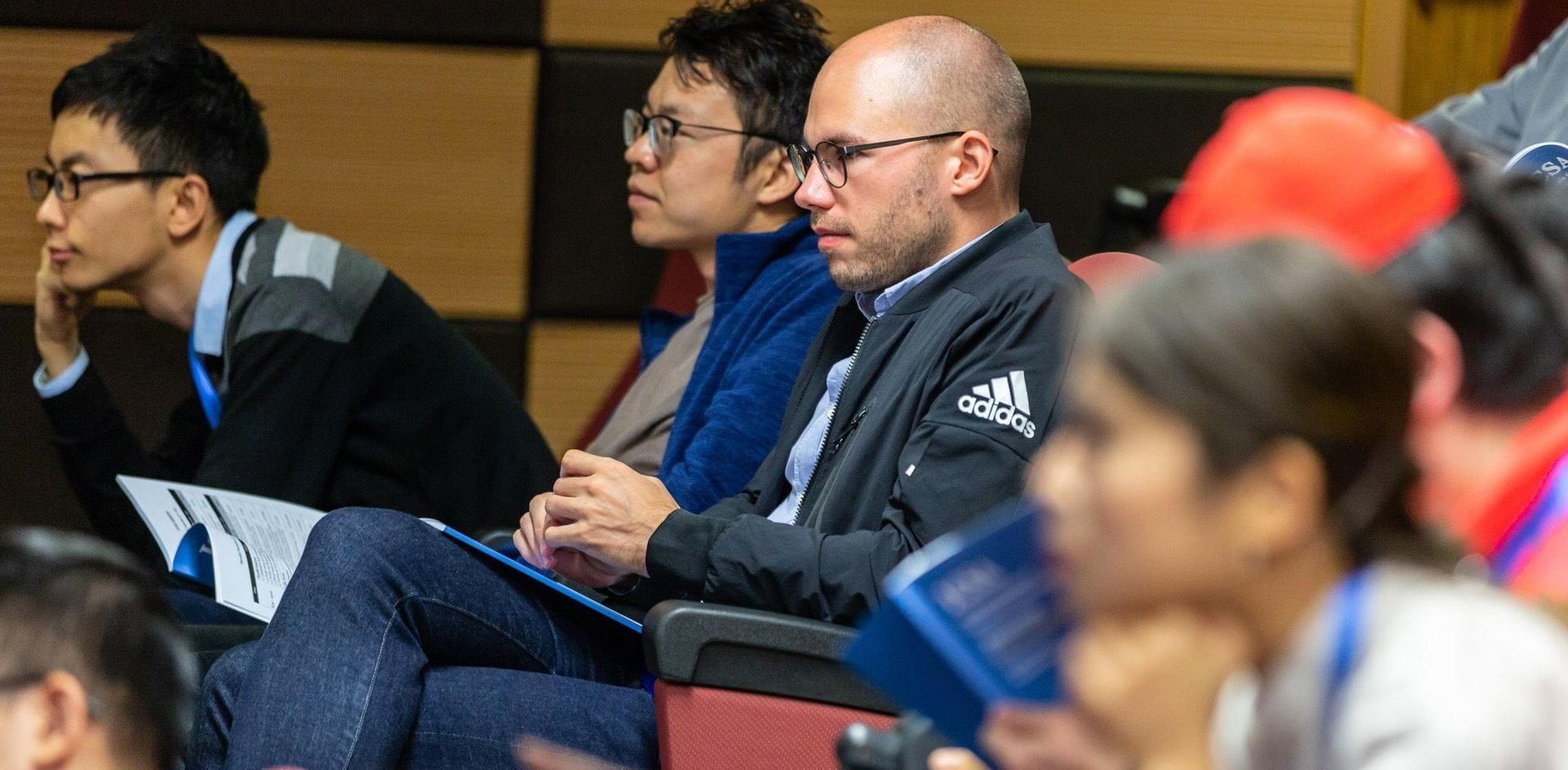
Embedded EthiCSTM @ Harvard Bringing ethical reasoning into the computer science curriculum
Topics at the Interface Between Computer Science and Economics (CS 236) – Spring 2018
First time reviewing a module? Click here.
Click ⓘ to access marginalia information, such as reflections from the module designer, pedagogical decisions, and additional sources.
Click “Download full module write-up” to download a copy of this module and all marginalia information available.
Module Topic: Interpretability and fairness
Module Author: Kate Vredenburgh
Course Level: Graduate
AY: 2017-2018
Course Description: “This is a rotating topics course that studies the interplay between computation and economics. Topics covered include but are not limited to electronic commerce, computational social choice, computational mechanism design, peer production, prediction markets and reputation systems. The class is seminar style and readings are drawn from artificial intelligence, theoretical computer science, multi-agent systems, economic theory, and operations research.”
Semesters Taught: Spring 2018
Tags
- interpretability (both)
- explainability (both)
- algorithms (CS)
- opacity (CS)
- explanation (phil)
- reasons (phil)
- rights (phil)
- obligation (phil)
Module Overview
In this module, we consider the ethics of interpretability. GDPR’s Article 15, 2f requirement that individuals be provided “meaningful information” about the logic of automated decisions. Legal scholars, politicians, and journalists have read GDPR as establishing a right to explanation, although not without pushback.
The module considers whether decision-makers ought to be required provide explanations of automated decisions, and, if so, what sort of explanations those should be. It first examines different reasons why one might say that that algorithms are not explainable. It then asks what underlying purpose explanation serves, such that there may be a right to explanation.
Connection to Course Technical Material
Two weeks of the course deal with interpretability. This module follows up directly on those weeks, asking why interpretability matters, whether it should be required, and whether the concept of interpretability is the same concept as explainability.
Goals
Module Goals
By the end of the module, students will be able to:
- Isolate properties of algorithms that make them opaque, or difficult to interpret or understand.
- Introduce students to different concepts of explainability, and how those concepts relate to interpretability.
- Discuss why explanations of algorithmic decisions are important.
- Brainstorm and examine technical and non-technical solutions to the problem of opacity.
Key Philosophical Questions
- When and why are algorithms opaque?
- What is the interest that could underly a right to explanation?
- What are technical and non-technical solutions to the problem of opacity in the form of specific rights protections?
Materials
ⓘ
This article distinguishes two different ways in which algorithms can be opaque: inscrutability, and non-intuitiveness. This distinction sets up the lecture’s discussion of what kinds of explanations decision-makers should be required to give of algorithmic decisions (if any), and whether it is technically feasible for them to provide these explanations. The article also argues for a particular solution to the problem of opacity, algorithmic impact statements, which are a very useful foil to the activity and discussion that ask students to brainstorm solutions.
- Barocas and Selbst (2018), “The Intuitive Appeal of Explainable Machines.”
Key Philosophical Concepts
ⓘ
Philosophers debate the precise definitions of these concepts. The Embedded EthiCS TA uses the following definitions:
Paternalism: the making of decisions for another person which are in their supposed best interest.
Autonomy: the capacity to think and choose for oneself.
Manipulation: a kind of influence that does not sufficiently engage the capacities for reflective and deliberative choice of the person being influenced.
Exploitation: when someone in a position of power takes advantage of a vulnerability in less powerful positions, in order to advance their own interests.
One might also include Sunstein and Thaler’s (the authors of nudge theory) concept of “Libertarian Paternalism” as a guide for permissible nudges. Unfortunately, they offer different definitions throughout their writings. According to Libertarian Paternalism, a nudge must: (1) respect the freedom of choice of individuals; and (2) be in the best interest of those nudged. (They are inconsistent on what “best interest” means: sometimes they say it is according to some objective measure other times they say “as judged by themselves.”).
- Reasons
- Explanation
- Interest-based accounts of rights
- Obligation
Implementation
Class Agenda
- An introduction to the problem of explainability: why is it important that algorithms are explainable?
- Discussion of why some algorithms are not interpretable, i.e., are difficult or impossible to understand.
- Discussion of what features of decisions in certain institutions give rise to a need for explanation: the exercise of power and distributed knowledge.
- Examine whether individuals are owed explanations directly, as a matter of respect, or whether individuals need explanations to be able to contest decisions, adjust their behavior to the rules, use their voice to influence institutions, etc.
- Brainstorming and discussion of technical and non-technical solutions, taking inspiration from Barocas and Selbst.
Sample Class Activity
Students are broken up into small groups and given short descriptions of real-world cases where an institution used an opaque decision-making algorithm. (Different groups receive different cases.) Groups are asked to: (1) identify what kind of explanation seemed to be required; and (2) brainstorm one technical and one non-technical solution that would enable decision-makers to give such explanations. The Embedded EthiCS fellow then leads a class-wide debrief, helping students to identify common themes in the groups’ answers.
Except where otherwise noted, content on this site is licensed under a Creative Commons Attribution 4.0 International License.
Embedded EthiCS is a trademark of President and Fellows of Harvard College | Contact us