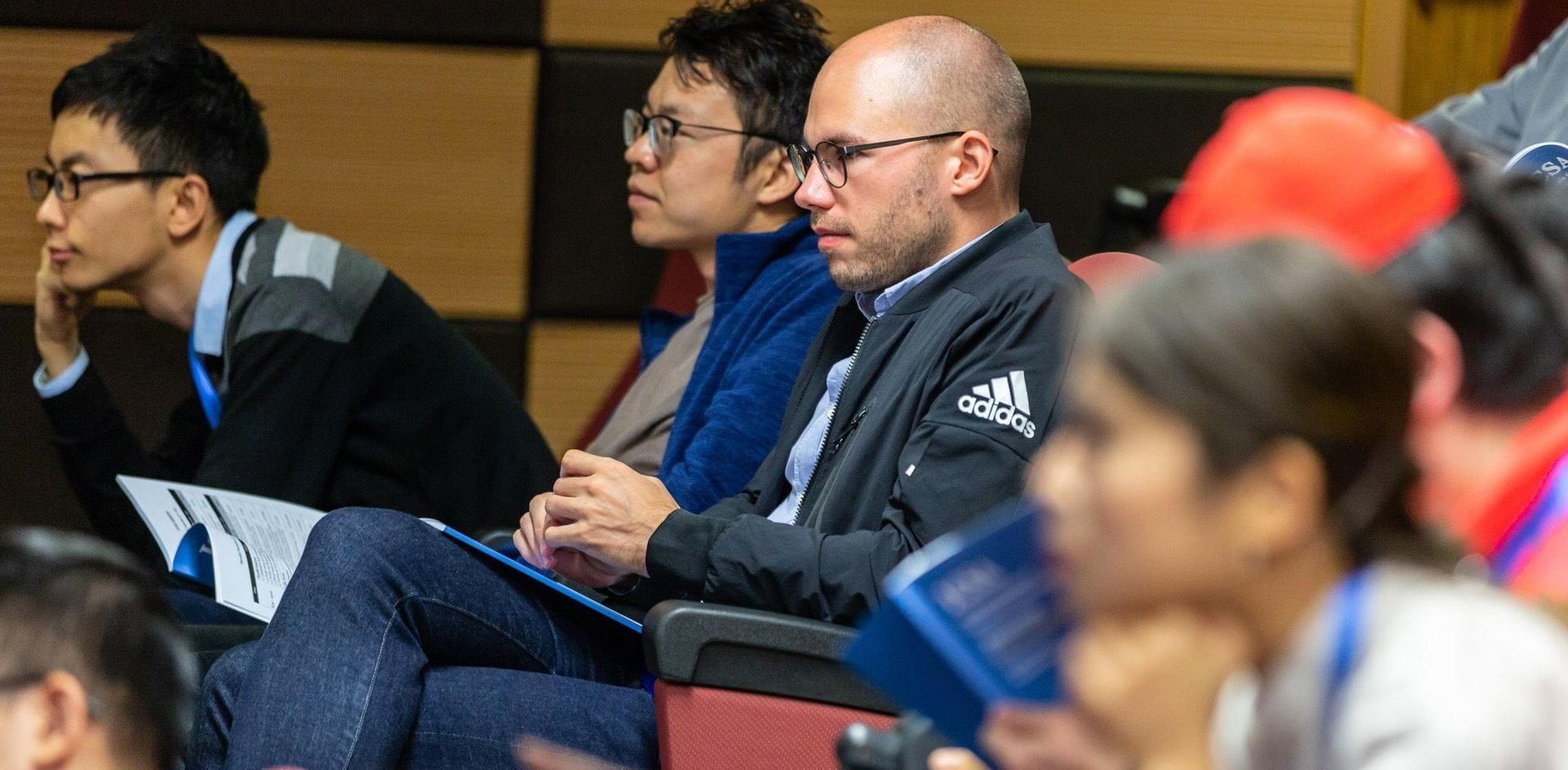
Embedded EthiCSTM @ Harvard Bringing ethical reasoning into the computer science curriculum
Intro to Data Science (CS 109A) – Fall 2020
First time reviewing a module? Click here.
Click ⓘ to access marginalia information, such as reflections from the module designer, pedagogical decisions, and additional sources.
Click “Download full module write-up” to download a copy of this module and all marginalia information available.
Module Topic: Injustice Ex(tra) Machina
Module Author: Elís Miller Larsen
Course Level: Introductory undergraduate
AY: 2020-2021
Course Description: “Data Science 1 is the first half of a one-year introduction to data science. The course will focus on the analysis of messy, real life data to perform predictions using statistical and machine learning methods. Material covered will integrate the five key facets of an investigation using data: (1) data collection – data wrangling, cleaning, and sampling to get a suitable data set; (2) data management – accessing data quickly and reliably; (3) exploratory data analysis – generating hypotheses and building intuition; (4) prediction or statistical learning; and (5) communication – summarizing results through visualization, stories, and interpretable summaries. Part one of a two part series. The curriculum for this course builds throughout the academic year. Students are strongly encouraged to enroll in both the fall and spring course within the same academic year.” (Course Description)
Semesters Taught: Fall 2019, Fall 2020, Fall 2021, Fall 2022
Tags
- statistical parity (CS)
- calibration (CS)
- error-ratio parity (CS)
- false positive rates (CS)
- false negative rates (CS)
- fairness (Phil)
- justice (Phil)
Module Overview
This module introduces the idea that big data can be unjust by unfairly representing the individuals the data is meant to be about. The injustice in data is further problematic because big data has become a predictive tool, not only representing individuals but making predictions about their future behaviors. The module focuses on one such predictive algorithm, the COMPAS (Correctional Offender Management Profiling for Alternative Sanctions) recidivism algorithm and data set, which generates predictions for prison inmate recidivism. This data set is a common example utilized in CS courses to discuss injustice because it illustrates the moral dangers of making predictions that lead to actual consequences for individuals since the algorithm is often used to determine the length of incarceration during pre-trial sentencing. The goal of this module is to help students identify how bias operates within the data set, and the data points where bias might arise, e.g., race, gender, class, and other social indicators. Model Cards are a recent development within research for ethical standards in CS. The students are provided with a practical assignment where they must create Model Cards that are outward facing “warning labels” for potentially biased data. These warning labels are designed to bridge the gap between designers and consumers of data. The assignment enables students to practice using tools that data scientists have begun to develop in order to mitigate bias and injustice in big data.
Goals
Module Goals
Key Philosophical Questions
ⓘ
These questions break down the three main goals of the module. The first two help students identify bias and injustice in data. And the third sets up the introduction of the practical application of Model Cards.
- What features of a data set might make it unfair?
- What features of a data set might make it unjust?
- How should we implement ethical standards into data science to mitigate injustice?
Materials
Key Philosophical Concepts
ⓘ
One virtue of focusing on these concepts is that it gives students a wider context for understanding how they are typically used within CS contexts, and how those uses may not correspond to typical meanings in other contexts.
- Fairness
- Transparency
- Accountability
- Justice
Assigned Readings
ⓘ
The first reading is a book chapter titled “Civilian Casualties: Justice in the Age of Big Data”. The author, a data scientist, outlines the way that big data can impact individuals and generate casualties that are unfair. This chapter is critical for students because it helps them make the connection between injustice in the world and injustice in data. O’Neil provides several examples where the data that is generated is simply a feedback loop of unjust practices in the real world. She argues that the data is then unfair to certain individuals because it continues to suppose that, for example, certain groups are prone to criminal activity or deserve higher loan rates. This chapter is an accessible introduction to the concepts of bias and injustice in big data. The second reading assignment builds on the concepts of the module by having the students learn about the statistical thresholds for fairness with an interactive game. Students are required to move the threshold for calibration in order to try to make the COMPAS data set fairer.
- Cathy O’Neil, Weapons of Math Destruction, Ch. 5
- Karen Hao and Jonathan Stray, “Can you make AI fairer than a judge?” (October 2019). MIT Technology Review.
Implementation
Class Agenda
- Overview of the ethical standards for CS
- Introduction of key philosophical and CS concepts and frameworks.
- Activity: Fill in a Model Card with the ethical considerations that would be apt as a “warning label” for users.
- Questions/Discussion
Sample Class Activity
Students were given a Model Card that is mostly filled out with the pertinent information for the COMPAS data set (sample model card below). The students are then asked to come up with a few ethical considerations or warnings in groups of 2 or 3 that should be included on the model card. Once this is completed the class re-groups to discuss the different warning labels students identified as relevant for the data set.
Module Assignment
No assignment was given for this module.
Lessons Learned
The module, as is, could be utilized for an advanced upper-level undergraduate or graduate course. For an introductory course, such as CS 109A, the module should be pared down. Students will need sufficient time to be able to connect the real-world consequences of the algorithm to the statistical components. For example, it will need to be emphasized that changing the threshold for fairness to make it more fair for one group, may make it less fair for others. It is also very important to take extra pedagogical care with teaching the COMPAS data set. Instructors should note that the data set depicts recidivism rates and the debate that ensues around the data set is about the disparity of recidivism rates between white and black defendants. This means that instructors must be aware of common pitfalls of teaching ethical topics that include race. It is important to avoid perpetuating stereotypical generalizations about racial groups that might isolate students or cause harm.
Except where otherwise noted, content on this site is licensed under a Creative Commons Attribution 4.0 International License.
Embedded EthiCS is a trademark of President and Fellows of Harvard College | Contact us